Mobile telecommunication networks and services are complex systems that are today planned and dimensioned by expert engineers in a static fashion,based on a limited set of local measurements and long-term statistics. In practice, however, the whole milieu is far from static, as subscribers are mobile by definition, and their communication activity patterns are strongly time-varying and location-dependent. In addition, the user traffic is increasingly generated by services that entail very different offered loads and requirements.
The strong contextual and content heterogeneity that characterizes the mobile traffic demand makes the sheer increase of capacity an inefficient strategy towards next-generation mobile networks. The capacity growth must be coupled with a much more efficient usage of the resources: next-generation mobile networks are expected to be flexible enough to adapt themselves to spatiotemporal variations and content diversity, and to do so in a timely and automated manner. This results in so-called cognitive mobile networks. These networks will run big data analytics on traffic measurements from in-network monitoring probes, so as to extract important knowledge about the current status of the system. This knowledge will then become a fundamental input for automatic network functions to engineer traffic and allocate resources in concertation with the needs of end users.
This vision primarily builds on the effective orchestration of network resources and services across all network levels. There is thus an ongoing substantial effort to define architectures for dynamic resource allocation,which all include orchestrator components in charge of taking automated decisions about resource reconfiguration. However, the dynamic resource management algorithms and policies that will run inside network orchestrators are to be entirely investigated and defined.
Designing orchestrator policies and algorithms entails a number of scientific and technological challenges: (i) Which data analytics shall drive cognitive networks? (ii) How to ensure scalable and real-time orchestration? (iii) How to integrate analytics into current virtualized network architectures? and (iv) Which are the gains of cognitive management?
To address the challenges, the CANCAN project targets the following objectives:
1) Collecting novel measurement datasets that describe mobile network data traffic at unprecedented spatial and temporal accuracy levels, and for different mobile services separately. The datasets will be gathered in an operational nationwide network.
2) Evaluating existing analytics for classification, prediction and anomaly detection within real-world high-detail per-service mobile network data,and tailoring them to the specifications of the management of resources at different network levels.
3) Demonstrating the integration of data analytics within next-generation cognitive network architectures in three practical case studies, i.e., (i) predictive and differentiated radio scheduling in virtualized Radio Access Network (vRAN), (ii) dynamic management of virtual machines and containers in Mobile Edge Computing (MEC), and (iii)dynamic Service Level Agreements and Class of Service generation for resource sharing and bottleneck mitigation. The integration will build on recent advances in architectures that enable Network Functions Virtualization.
The CANCAN project brings together four partners: Thales advanced studies (Thales), the Agora team of the Inria Rhone-Alpes center (Inria), the ROC team of CNAM, Paris, and the SENSE department of Orange Labs (Orange).
The project runs from Jan. 2019 to June 2022.
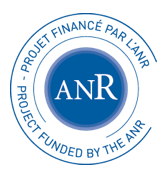